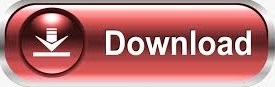
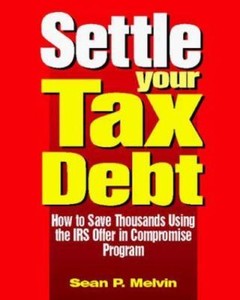
Section 5.3 of my edition of your text explains a standard situation that leads to right truncation: when you enroll participants into a study only after they have developed some disease. That applies to the entire sample at hand. From that perspective in the example case, you have to treat the age values of the observations you have as left-truncated, as the sample provides no information about ages below the threshold. Your professor's emphasis is on how you analyze the data that you do have. Wikipedia puts it nicely:Ī truncated sample can be thought of as being equivalent to an underlying sample with all values outside the bounds entirely omitted, with not even a count of those omitted being kept. What you have is a sample of non-truncated observations. But you don't have those truncated "observations" to work with at all. Yes, a truncated "observation" is one that is unavailable to the study because its value is out of range. I think that the apparent discrepancy between the text and your professor has to do with the truncated (and thus missing) "observations" versus the implications for how you handle the data that you do have.

I am having a hard time coming up with a natural example of right truncation? Does anyone have a good one? Which one is the correct one? If there are different conventions, then which one is the most common one?Īlso, the above example is an example of left truncation. So from what I understand the book claims truncation is a local property, while my professor claims that it is a global property (in the sense that the data set is truncated). In the same example ALL observations are truncated since they have survived beyond age 60 and are only in the study because of this condition. Professor: Truncation occurs when observations are only in the study given some condition. Hence dead people younger than 60 are truncated in this study. For instance if we are modelling time to death but only looking at people at retirement homes, we miss people under the age threshold (say 60) to be admitted to the retirement homes. Now here is where the book and my professor's explanations differ a bit:īook: A truncated observation is one that has been left out due to some restriction of the study. So the main difference between censoring and truncation is whether or not we have access to all potential observations or not, is it not? Hence censoring is (1) a local property in the sense that we talk about a observation being censored, and (2) censored observations are in the study.įrom my current understanding, truncation on the other hand occurs when the study is conducted in such a way that some potential observations are left out. Moeschberger.Īlthough I like the book in general, I don't really understand the way truncation is explained in the book and my professor's explanation hasn't helped too much either - and what is worse they seem to conflict a little bit in my head.įrom my current understanding censoring in survival analysis occurs when either the event we are trying to model the time to has already been triggered for an observation in the study ( left-censoring) or if for the duration of the study we don't see the event occur for an observation ( right-censoring). Note: most of these estimates are provided by R and SAS, so only minor hand calculations are necessary.I am taking a course in survival analysis where we follow the book "Survival Analysis: Techniques for Censored and Truncated Data" by John P. Ob 90 B blomoly (f) Using a range of 1 to 104 weeks, find the lower and upper bounds of the 95% Hall- Wellner log-transformed confidence band at 26 weeks. (e) Construct the 95% log-transformed (pointwise) confidence interval for the survival function at 26 weeks. (d) Estimate for the median time to death and find a 95% confidence interval for median survival time. We are using the subset consisting of diploid patients only. Type ?tongue in the console after loading the KMsury library to see it. (c) Estimate i r the mean time to death and find a 95% confidence interval for mean survival time. d errors - clo in R Co in or SAS SA (b) Estimate the cumulative Hazard rates and their standard errors through the 13th week using the Nelson-Aalen estimator. (a) Estimate the survival function values and their standard errors.

Consider the diploid tumor patient data in Table 1.6, Section 1.11 on page 127.
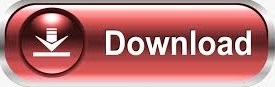